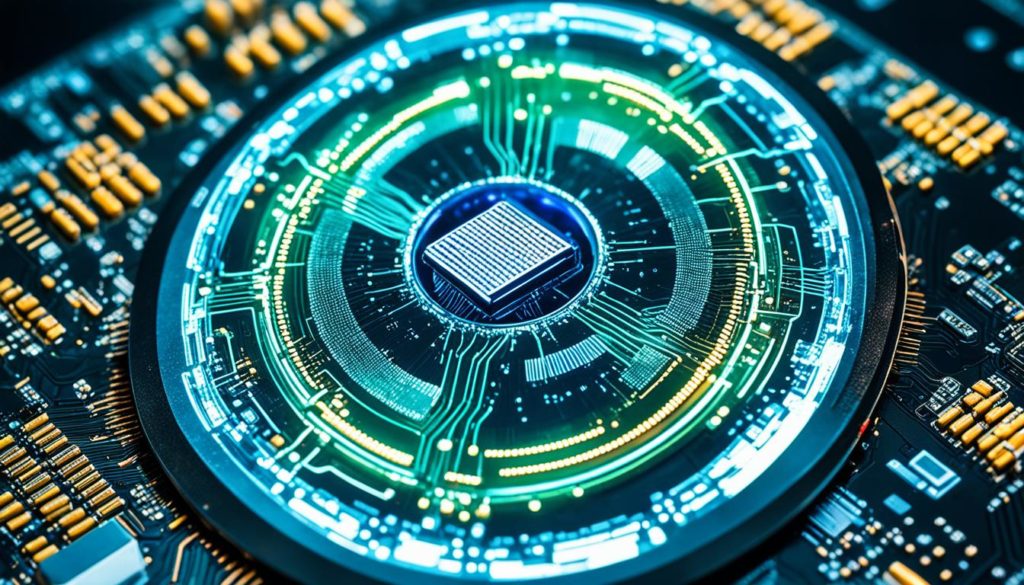
Adversarial AI manipulation could trick self-driving cars into misreading signs, leading to accidents. This happens as AI gets more involved in our lives, raising the stakes for risks of adversarial attacks. Such risks don’t only threaten digital safety but also physical well-being. Our discussion highlights the rise of adversarial AI, its dangers, and the latest defensive strategies to enhance artificial intelligence security. Making AI systems reliable is crucial in today’s world.
Key Takeaways
- Adversarial AI poses substantial risks to AI systems, impacting both digital and physical domains.
- Understanding different types of adversarial attacks is crucial for AI security.
- Proactive defensive strategies can enhance the robustness of AI models against malicious manipulation.
- Collaboration among stakeholders is vital for developing comprehensive solutions to adversarial threats.
- Addressing ethical considerations is essential in reinforcing trust and accountability in AI systems.
Understanding Adversarial AI and Its Implications
Adversarial AI uses techniques to trick or change AI systems. These can range from small changes in data to complex tricks. Knowing about these is key because they can harm many areas. These include things like image spotting, keeping data safe, and self-driving tech. Adversarial AI’s power to break into machine learning, particularly deep learning networks, threatens AI’s reliability and how it performs.
What is Adversarial AI?
Adversarial AI exposes weaknesses in learning models of AI. It makes slight yet smart changes to make AI fail in its tasks. These tricks are hard to spot and fix. To fight these issues, experts are working on ways to make AI tougher. Methods like adversarial training and defensive distillation are being used. This ongoing fight shows how growing and changing adversarial AI is.
Types of Adversarial Attacks
Different types of attacks exist, like evasion, poisoning, and stealing models. Evasion changes inputs to mislead AI, often hurting image recognition and security. Poisoning messes up training data, damaging the AI’s learning. Attacks are split into white-box and black-box, depending on what attackers know. White-box attackers know the model well. Black-box attackers guess because they have less info.
Real-World Examples of Adversarial Attacks
Adversarial AI has big real-world effects. It’s seen in messing with cars that drive themselves and systems that recognize faces. In 2021, some made facial rec software fail using makeup. By 2023, they tricked AI chatbots into wrong answers with small prompt changes. These examples show why it’s important to keep up defenses and stay alert.
Adversarial AI is getting more attention for its ability to mess with AI. Understanding it fully and defending against it is crucial. Techniques evolve, from adversarial training to always checking on systems. Staying ahead is key to keeping AI safe and trustworthy.
For an in-depth exploration, learn more about adversarial attacks on AI and Machine, delve into adversarial AI developments, and explore insights on adversarial AI.
Challenges and Risks Associated with Adversarial AI
The growth of artificial intelligence brings new challenges. It’s important we deal with the risks of adversarial AI. This means we need a well-rounded plan to keep AI safe, honest, and protected from attacks.
Robustness and Security of AI Models
AI weaknesses can mess with how AI systems work. They need strong defense strategies. Making AI able to deal with attacks is key for keeping important areas safe. Developers should focus on stopping bad guys from breaking into AI models.
Trust and Accountability in AI Systems
For people to trust AI, it has to be accountable. The danger with adversarial AI is it makes things uncertain and risky. Having clear AI operations and telling people about dangers can help build this trust. It’s important that AI works well and that someone takes responsibility when it doesn’t.
Legal and Regulatory Frameworks
The laws around AI are trying to keep up with new threats from adversarial AI. We need laws that make sure AI is ethical and doesn’t harm people. Lawmakers have to keep up with technology to make AI safe and ethical.
Challenge | Impact | Mitigation |
---|---|---|
AI Vulnerabilities | Compromises model integrity | Implement adversarial risk mitigation measures |
Accountability and Trust in AI | Reduces public confidence | Ensure transparency and clear communication |
Regulatory Aspects of AI | Legal and ethical compliance | Develop comprehensive legal frameworks |
Adversarial AI: Identifying Solutions and Mitigation Strategies
We’re in a battle with adversarial AI, where finding the right defense matters. We’ll look at adversarial training, defensive distillation, input sanitization, and anomaly detection. It’s key to work together to make AI safer.
Adversarial Training
Through adversarial training, AI models learn from tough examples. This makes them smart at spotting and stopping shady inputs. The technique helps AI fight off sneaky attacks, making them stronger in the real world. A NIST report says this approach is vital but not enough, urging for more breakthroughs.
Defensive Distillation
Defensive distillation helps AI models make less mistakes when tricked by tiny data changes. It’s an extra shield against attackers. Yet, it’s not a complete solution alone. We need a mix of methods.
Input Preprocessing and Sanitization
Input sanitization protects against attacks by cleaning up data first. It reduces the chance of AI being fooled or poisoned. Using anomaly detection, unusual data gets caught early.
Anomaly Detection and Monitoring
Anomaly detection and constant watch are crucial for AI safety. By always checking the data and AI behavior, threats can be caught fast. Model monitoring helps find odd AI reactions, leading to quick action against enemies. Strong anomaly detection tools are a must for AI’s trustworthiness.
Collaboration among Stakeholders
Teamwork is key for AI security. Researchers, coders, and policymakers need to join forces. Together, they share knowledge and create solid defenses. This way, AI can stand up to smart attacks.
Ethical Considerations in Adversarial AI Defense
In the world of responsible artificial intelligence, finding the right balance is key. We must balance strong security with protecting privacy when dealing with ethics in AI defense. Fair AI systems can accidentally make biases worse. So, we need to make sure our defenses respect data privacy.
Protecting privacy is at the heart of trustworthy and fair AI. Unauthorized access to personal data is a major risk that we must watch closely. At the same time, it’s vital that AI models are transparent. If they’re not, their decisions could cause harm without anyone understanding.
Ethical AI use also requires thinking about the dual purposes of research. The advances that strengthen AI could also be turned against us by bad actors. This is why putting ethical and regulatory systems in place is crucial. They help make sure AI is used right and prevent misuse.
Working with policymakers, industry heads, and community groups is crucial for ethical AI growth. Forming partnerships between public and private sectors can boost research and share valuable knowledge. These efforts are key to fight AI threats and add ethical considerations to AI defense strategies.
As we advance AI defenses, we need to keep an eye on ethics. Moving towards responsible AI involves constant attention and adjustments. This ensures our steps forward are fair and responsible.
Conclusion
In wrapping up our detailed look at adversarial AI, we see it’s key to use multiple strategies. This includes mixing tech safeguards, ethics, and teamwork. Together, they create a strong defense for AI security.
The methods we talked about, like training AI to recognize attacks and spotting unusual activities, are crucial. Making sure machine learning is safe is a must. As threats grow, our AI defenses must be up to the challenge.
It’s up to all of us to make sure AI technology grows in a safe and secure way. By focusing on cutting-edge security and ethics, we’ll ensure AI benefits us all. Our dedication to these goals will help AI blend into our lives safely, without harming trust or security.
FAQ
What is Adversarial AI?
Adversarial AI aims to trick AI systems to cause harm. It uses methods like adversarial examples, data poisoning, and evasion attacks.
What are the types of adversarial attacks?
Types of attacks include adversarial examples, data poisoning, model stealing, evasion attacks, and adversarial reprogramming. Each targets AI systems differently.
Are there real-world examples of adversarial attacks?
Yes. Attacks have hit areas like computer vision, cybersecurity, and autonomous systems. They often use subtle manipulations or inputs that trick security measures.
How do adversarial attacks affect the robustness and security of AI models?
These attacks show AI models’ weaknesses, hurting their robustness and security. This is critical in areas where safety matters a lot, highlighting the need for tougher AI models.
What role does trust and accountability play in AI systems under threat from adversarial attacks?
Adversarial attacks seriously damage trust and accountability in AI systems. They threaten system integrity and reliability, lowering public trust and raising questions about who is responsible when AI fails.
What are the legal and regulatory frameworks addressing adversarial AI?
Laws and rules for adversarial AI are being developed. They aim to create standards and guidelines to lower these risks, assure accountability, and protect data privacy.
What is adversarial training?
Adversarial training boosts AI models’ toughness by exposing them to adversarial examples during training. This helps them resist attacks better.
How does defensive distillation work?
Defensive distillation trains AI models to ignore minor changes. This improves their strength against adversarial attacks.
What is input preprocessing and sanitization?
Input preprocessing and sanitization clean and normalize data before it enters AI systems. This reduces the chance of adversarial examples messing up model performance.
How can anomaly detection and monitoring aid in defending against adversarial attacks?
Anomaly detection and monitoring spot odd patterns and behaviors in AI systems early. This helps stop adversarial attacks before they cause trouble.
Why is collaboration among stakeholders important in countering adversarial AI?
Teaming up researchers, developers, and policymakers is key. They work together on solutions for technical, ethical, and legal challenges of adversarial AI.
What are the ethical considerations in adversarial AI defense?
It’s ethical to balance security and privacy, fix biases, keep things transparent, and handle dual-use research risks that could be exploited.
Future App Studios is an award-winning software development & outsourcing company. Our team of experts is ready to craft the solution your company needs.