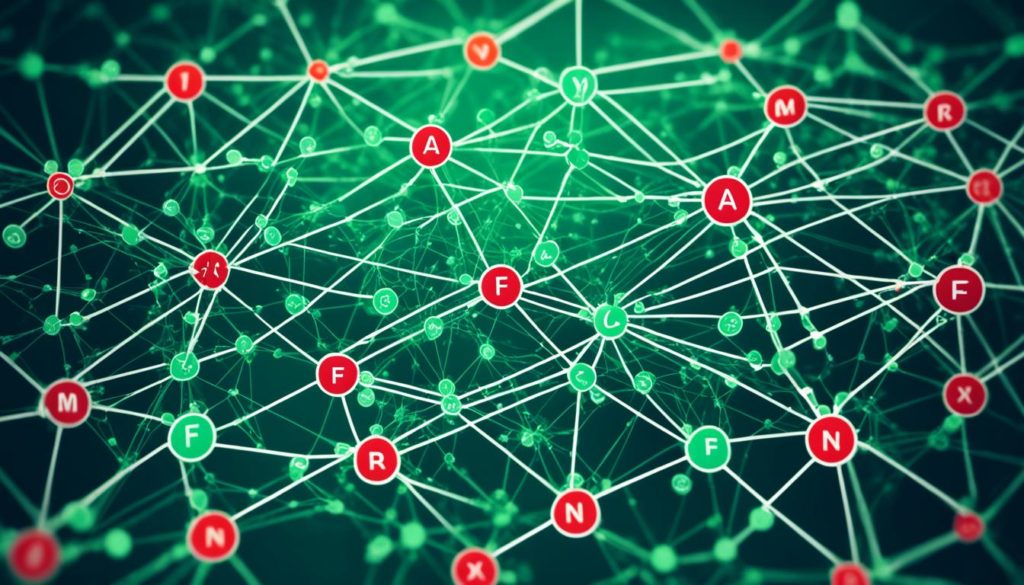
Did you know that around 426,000 credit card fraud cases were reported in 2023? This number is a bit less than in 2022 but still 53% higher than in 2019. Such a big increase shows we need better security fast. That’s where machine learning fraud detection comes in. It’s a new, smart way to fight financial crimes.
Machine learning algorithms are super fast and accurate. They can look through lots of data to find signs of fraud. Unlike old systems that follow set rules, machine learning models get better as they learn from new information. This ability to adapt matters a lot. It helps spot and stop new fraud tricks early.
When we add machine learning to other smart tech like NLP and computer viewers, fraud detection gets even better. These AI tools allow for watching all the time and reacting fast to dangers. This protects our money and everyone involved from fraud.
Yes, using artificial intelligence to stop fraud means thinking about data quality and following rules. Yet, about 17% of places already use AI and machine learning for this. So, it’s clear that using smart analytics to find fraud isn’t just a future idea. It’s happening now and it’s working.
Key Takeaways
- Machine learning algorithms can rapidly analyze extensive datasets to pinpoint fraudulent activity.
- These systems adapt and improve over time, learning from new data and experiences.
- AI-driven technologies enable real-time fraud detection and quick response mechanisms.
- Combining machine learning with NLP and computer vision enhances fraud detection capabilities.
- Implementing machine learning in fraud prevention mandates close attention to data forging and regulatory compliance.
The Importance of Machine Learning in Modern Fraud Detection
Digital transactions are everywhere now. This makes the weaknesses of old fraud detection ways more obvious. Machine learning steps in with a fresh and flexible strategy. It is crucial for fighting clever and ever-changing online threats.
It quickly works through huge amounts of data. This way, it spots complex fraud attempts better than old systems. The term real-time fraud detection highlights machine learning’s ability to quickly adapt. This fast adjustment is vital for outsmarting fraudsters who are always finding new tricks. Also, the rise in online threats makes machine learning’s fast and forecasting powers a must-have.
Why Traditional Methods Are No Longer Sufficient
Old-school fraud detection depended a lot on fixed rules and unchanging models. Sadly, these can’t keep up with today’s clever fraudsters. As these crooks use more advanced tricks, the old methods can’t catch up fast enough. On the flip side, machine learning models for spotting fraud are always getting smarter. They learn from new information and get better at finding fraud.
The Rise of Cyber Threats in Online Transactions
Online shopping and payments are more common than ever. This has led to a big increase in online scams. The systems we used before, meant for simpler scams, now fall short. But, machine learning models for finding fraud use big data to quickly spot strange activities. They learn from new patterns of fraud, giving us a strong tool to keep digital payments safe.
Comparison Aspect | Traditional Methods | Machine Learning Models |
---|---|---|
Adaptability | Low | High |
Speed of Detection | Slow | Real-time |
Accuracy | Moderate | High |
Data Handling | Limited | Vast |
How Machine Learning Detects Fraud in Real-Time
In today’s digital world, machine learning is key to better fraud detection. It uses smart algorithms to review tons of data quickly, finding odd patterns that old methods might miss.
Anomaly Detection: Spotting the Outliers
Anomaly detection is vital in fighting fraud. It looks closely at transactions to find anything that doesn’t match normal patterns. For example, a sudden spike in someone’s spending could signal a problem. With machine learning, these signs are caught fast, making things safer.
Building Predictive Models for Fraud Prevention
We also build predictive models to stop fraud before it happens. These models look at various aspects of transactions. They use advanced techniques, including anomaly detection, to score the risk of each deal. This way, we have a strong system to identify fraud as it tries to happen. The mix of anomaly detection and predictive modeling makes a powerful and exact way to reduce risk in the huge online transaction world.
Fraud Detection Algorithms: Techniques and Tools
Fraud detection uses many machine learning models, each made for different fraud-fighting tasks. These models help spot fraud more correctly and keep up with new fraud tricks. They provide a strong defense for businesses.
Supervised Learning Models
Supervised learning models are key in spotting known fraud ways. They train on data that’s already tagged to sort different fraud types. For instance, places like JPMorgan Chase use supervised learning to tell real credit card buys from fake ones. Supervised learning’s big plus is using past data well. Yet, it needs high-quality, tagged data to work best.
Unsupervised and Self-Supervised Learning Models
Unsupervised and self-supervised learning models work without tagged data. They’re great at finding hidden fraud patterns. This is crucial for catching fraud that’s never been seen before. Companies like Google and Facebook lead the way in self-supervised learning. This improves spotting odd things in huge data sets. These models are flexible and give a wider safety net against fraud.
Using these different algorithms with tech like natural language processing (NLP) and computer vision makes fraud spotting even better. Here’s how these approaches compare:
Algorithm Type | Data Requirements | Strengths | Limitations |
---|---|---|---|
Supervised Learning | Labeled Data | High Accuracy, Task-Specific | Dataset Dependent, Requires Quality Labels |
Unsupervised Learning | Unlabeled Data | Detects New Patterns, Flexible | Complexity, May Overfit |
Self-Supervised Learning | Minimally Labeled | Adaptive, Efficient Data Use | Requires High Computational Power |
Fraud Detection with Machine Learning
Machine learning, including deep learning, changes how we tackle fraud detection. It’s different from old systems that needed constant manual work. Now, we have smart fraud detection tools. They learn on their own and get better at spotting new threats.
Our tools keep learning from huge amounts of data. They become more accurate and efficient. This is important to catch complex fraud that old methods might not see.
With deep learning, businesses can look closely at transaction data. They can spot unusual patterns and possible threats accurately. This not only makes things safer but also builds more trust with customers.
Let’s look at some important points about using machine learning for fraud detection:
- Scalability: These models handle growing data without trouble.
- Real-time Analysis: They can spot fraud right away, leading to quicker action.
- Adaptability: The algorithms get better over time, catching new types of fraud.
Adding deep learning to fraud detection provides a strong and flexible way to manage risk. In today’s digital age, using these advanced methods is essential. They help businesses stay ahead in stopping fraud.
Benefits of Implementing Machine Learning in Fraud Detection
Using machine learning for fraud detection brings many benefits that change how we fight fraud. It uses advanced algorithms and computing to spot and stop fraud more accurately and quickly.
Adaptability to New Fraud Patterns
Machine learning’s ability to adjust to new fraud trends is a key advantage. It can quickly learn from new data, keeping our defenses strong against new threats. GBG Alert, for example, can find identity theft and other frauds with up to 89% accuracy. This fast learning helps us stay one step ahead of fraudsters.
Reduction in Human Resource Requirements
The need for human checks goes down, thanks to AI and ML in fraud detection. This makes finding fraud cheaper and more efficient. For instance, GBG’s systems find 80% more fraud than older methods, easing the load on people. AI also works 24/7, letting experts focus on important tasks.
Machine learning also cuts down false alarms by at least 40 percent. This means a more trustworthy system. It secures transactions and makes the user experience better by avoiding unnecessary checks. With global online fraud losses possibly hitting $48 billion by 2023, having an effective and affordable fraud detection system is crucial.
Case Studies: Success Stories in Machine Learning Fraud Prevention
Machine learning has changed how we stop fraud, especially in banks. By using machine learning for fraud detection, fewer scams happen. This makes money matters safer for everyone. Here are some AI success stories that show how effective it can be.
Real-World Applications
Online fraud costs billions every year. That’s why solutions like Amazon Fraud Detection are so important. They’ve been fighting fraud for 20 years and help stop it fast.
Aella Credit is a great success story in markets that are just starting to grow. They use Amazon Rekognition to check identities better and faster. Then there’s FlightHub Group, whose fraud dropped a lot thanks to Amazon Fraud Detector.
In tough times, companies like ActiveCampaign stand out. They used detailed transaction data to fight off phishing in 2020. Their story shows how adaptive and quick machine learning can be in detecting fraud.
Impact on Financial Institutions
Machine learning has made a big difference in fighting fraud at financial institutions. A study saw that 62% of big banks saw more financial crimes, costing billions. Machine learning for fraud detection gives them a way to fight back.
Banks with big assets lost around $120 million to attacks in 2022. Machine learning helps catch these through methods like checking for odd behavior. Banks like JPMorgan and Bank of America have seen great results. This shows the big impact it can have.
These AI stories are just the start. They show other businesses how to better find and stop fraud. With advanced AI, having safe and stable finances is an achievable goal for everyone.
Challenges in Deploying Machine Learning for Fraud Detection
Deploying machine learning for fraud detection comes with challenges. It is vital to ensure the data is of high quality. This ensures the accuracy and trustworthiness of ML models. Also, it’s important to keep the models clear and understandable.
Data Quality and Model Interpretability
Fraud detection often deals with only a small number of customers trying to cheat. This creates a challenge in categorizing data accurately. ML models must be updated often to keep up with new fraud methods. This keeps them working well and efficiently.
Creating features for detecting fraud takes a lot of expert time. These features have to be well thought out. Using techniques like explainable AI helps in understanding why certain transactions are seen as suspicious.
Regulatory Compliance
AI systems for fraud detection must follow laws like GDPR and CCPA to protect user data. They also need to be good at spotting likely frauds. For example, keeping data safe is key in fraud detection. Using the human-in-the-loop method helps fix data categorizing problems and speeds up feature finding. It does this by getting humans to help spot new fraud trends for the models. To understand the significance of regulatory compliance in AI better, look at more resources.
Effectively using machine learning in fraud detection means tackling these complex issues. Addressing them is crucial for success and for gaining trust widely.
Conclusion
Machine learning has truly changed how we detect fraud, making transactions safer. Companies using this technology are stronger in fighting cyber threats. This shows the importance of machine learning in defending against fraud.
Machine learning detects fraud instantly and learns new fraud patterns. Yet, there are hurdles like data quality and model understanding. But with careful planning, we can overcome these challenges.
Looking ahead, machine learning will keep getting better, protecting our financial systems. By using advanced detection methods, we make the digital world safer for all.
FAQ
What makes machine learning an effective tool for fraud detection?
Machine learning can look closely at financial data and see patterns. It spots fraud as it happens and learns new fraud types. This way, it’s better than old, rule-based methods.
How do fraud detection algorithms work?
Fraud detection uses different kinds of machine learning. These include supervised and unsupervised models. They find odd activities, make predictions, and update to fight new fraud tricks.
Can machine learning handle the rise of cyber threats in online transactions?
Yes, machine learning works well against complex cyber threats. It handles lots of data and changes as needed. It keeps online payments safe.
What techniques does machine learning use to detect fraud in real-time?
Machine learning looks for unusual patterns and predicts fraud chances. These steps help catch suspicious actions quickly.
How does deep learning contribute to fraud detection?
Deep learning lets models learn from data on their own. It spots complicated fraud attempts better than older ways. This makes it a strong option for catching fraud.
What benefits do businesses gain by implementing machine learning in fraud detection?
Businesses keep up with new fraud types easily with machine learning. It cuts down on the need for lots of workers, makes fewer mistakes, and saves money. All this makes financial transactions safer.
What are the real-world applications of machine learning in fraud detection?
Machine learning has helped stop fraud in many fields, especially in banks. It has lowered fraud, found more fraud cases, and handled big data. This leads to safer finance systems.
What challenges must be addressed when deploying machine learning for fraud detection?
It’s key to have good data and clear models and follow laws like GDPR. Machine learning must protect personal data while finding fraud well.
Future App Studios is an award-winning software development & outsourcing company. Our team of experts is ready to craft the solution your company needs.