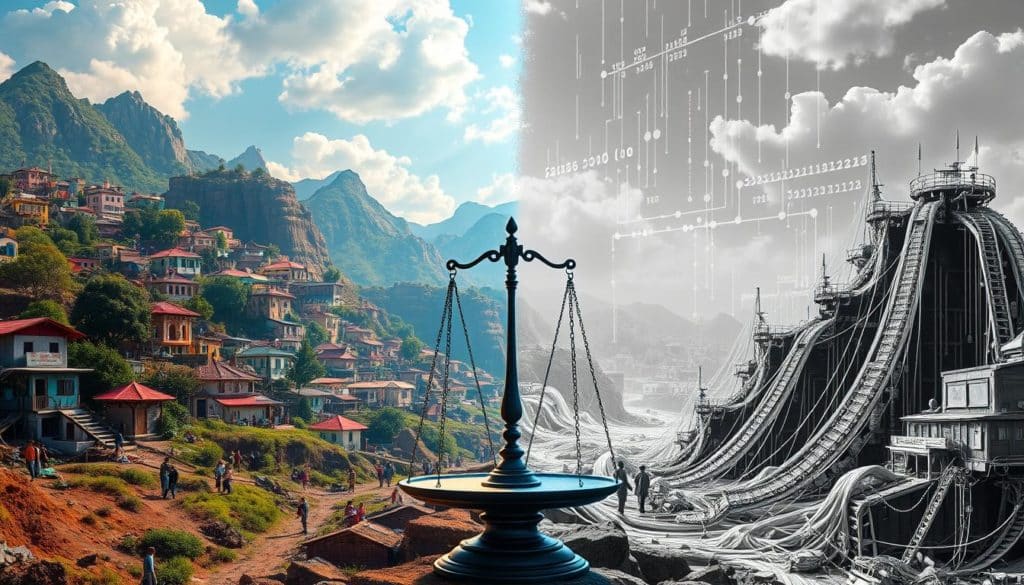
Did you know AI bias could cause legal problems, non-compliance, and harm a company’s reputation? This fact shows how important it is to tackle biases in AI. As AI becomes more common in our lives, it’s key to see how it affects us and society.
AI bias happens when AI systems are flawed due to bad data or algorithms. This can lead to unfair outcomes, making things worse for certain groups. For example, IBM is working hard to fix these issues with strong AI rules. They focus on being open, fair, and having humans check AI’s work.
More and more, people see the need to fix AI bias as AI gets used everywhere. This includes important areas like healthcare, finance, and law enforcement. Leaders need to keep up with AI to avoid unfairness and make sure AI is fair and just.
Working together with AI is key to solving these problems. This means humans and machines team up to spot and fix biases. Also, having diverse teams and listening to those affected is crucial for spotting and fixing biases.
Key Takeaways
- AI bias can lead to significant legal and reputational risks for businesses.
- Recognizing and addressing machine learning biases is essential for ethical AI deployment.
- Collaboration between humans and machines is crucial to mitigate AI bias.
- Diverse AI communities play a key role in identifying and correcting biases.
- Organizations like IBM are pioneering AI governance to ensure fairness and transparency in AI systems.
What is AI Bias?
AI bias, also known as machine learning bias or algorithm bias, happens when an algorithm shows unfair results. It’s important to understand the types of bias to reduce their harm. By looking at where bias comes from and how it affects us, we can make AI fairer for everyone.
Definition of AI Bias
AI bias shows up as unfair results from AI systems that reflect human biases and social inequalities. This bias can be either obvious, from biased data, or hidden, from how the data was gathered. For example, a US hospital’s algorithm favored white patients over black, based on past healthcare costs.
Sources of AI Bias
AI bias comes from many places in the AI process. Mistakes in data collection, flawed algorithms, and biased labeling are all culprits. Common biases in AI include stereotyping, priming, and confirmation bias. To fix this, we need good AI governance and policies. Techniques like data cross-validation and model simplification can help reduce bias.
For more on how to spot and fix AI bias, check out this resource on AI bias.
Impact on Marginalized Groups
The AI impact on marginalized communities is significant. If not addressed, AI bias can make things worse, limit job chances, and keep discrimination alive. For example, Amazon’s hiring tool showed bias against women by looking down on resumes with female names. Racial biases in police tools also unfairly target some groups. To fix this, AI needs to be trained on diverse data and checked regularly for fairness.
Examples of AI Bias in Real Life
AI bias is a big problem in many areas, causing real-world issues. By looking at examples, we can understand the problems and find ways to fix them.
Healthcare
In healthcare, biased algorithms can lead to unfair treatment. For example, a US healthcare algorithm showed an 80% bias against black patients. This affects how doctors diagnose and treat patients, especially if the data doesn’t include diverse groups.
A 2023 study found that most skin cancer image datasets lack information on ethnicity or skin type. This can lead to wrong diagnoses, especially for people with darker skin.
Applicant Tracking Systems
AI in hiring can also be biased. Amazon’s hiring tool, for instance, favored male applicants. This is because it was trained on data that showed men were more likely to apply for tech jobs.
This bias makes it hard for women to get tech jobs. It shows we need to make sure hiring tools are fair and regularly checked.
Online Advertising
Online ads also show gender bias. Studies found that job ads on Facebook are often shown to men, even if they’re for jobs that could be done by women. This limits job opportunities for women and keeps old gender roles alive.
UNESCO suggests using AI that doesn’t favor one gender. This could help change how we see and treat different genders, even in things like voice assistants.
Image Generation
Tools like Midjourney have been criticized for their bias. They often make images of people looking young, which can be seen as ageist. The Lensa AI app was also found to make avatars of women in a sexualized way.
A 2023 study found that AI models can be biased in age, gender, and how they show emotions. This shows we need to work on making AI fairer.
Predictive Policing Tools
Predictive policing tools can also be biased. The COMPAS algorithm in the US was found to predict higher recidivism rates for black offenders than white ones. This can lead to unfair treatment and even wrongful convictions.
It’s important to regularly check these tools to make sure they’re fair. McKinsey has suggested ways to do this.
For more on AI bias, check out this article.
Sector | Example | Impact | Mitigation |
---|---|---|---|
Healthcare | Biased diagnostic algorithms | Skewed medical care for minorities | Inclusive datasets, audits |
Recruitment | Amazon’s biased hiring algorithm | Reduced opportunities for women | Bias-free training data, diverse teams |
Online Advertising | Gender-skewed job ads | Limited job visibility for women | Gender-neutral AI advocacy |
Image Generation | Midjourney’s age and gender biases | Reinforced stereotypes | Debiasing strategies |
Policing | COMPAS algorithm bias | Unfair treatment of black offenders | Algorithm audits, diverse data |
How AI Bias Affects Society
Algorithmic bias in AI systems has big effects on society. Looking at these impacts helps us see the bigger picture. We’ll explore the economic, social, and trust issues in technology.
Economic Implications
The economic effects of biased AI are big. Bias in algorithms can block fair competition, making job chances worse for some. For example, AI in hiring might unfairly pass over certain groups.
As AI gets into more industries, fixing these issues becomes more urgent. Biased AI can also deny loans to minority groups, making the wealth gap bigger.
Social Consequences
The social effects of AI bias are also big. It can make divisions worse and hurt social unity. AI systems that show bias can leave out minority groups, leading to unfairness.
For instance, studies found gender biases in language models, making stereotypes worse. Biased AI in schools can also hurt kids from poor backgrounds, keeping them from getting ahead.
Trust in Technology
Trust in AI technology is a big issue when biases are seen. AI needs to be fair to be trusted. When it’s not, people lose faith.
This is especially true in healthcare, where wrong AI decisions can harm patients. Doctors might follow AI advice without checking, making their choices less accurate. Fixing trust in AI is key for it to work right.
Mitigating AI Bias
AI Governance and Policies
AI governance strategies are key to avoiding bias. They cover rules, trust, openness, and human checks. For example, using diverse data sets in policies can help a lot. Companies like IBM are working hard to make AI fair and unbiased.
Technical Solutions
There are many ways to fight AI bias. Methods like fairness checks and learning from mistakes are important. It’s crucial to use these methods at every stage of AI development. The AI Fairness 360 toolkit is a free tool to help find and fix AI bias.
Human-in-the-Loop Systems
Human-in-the-Loop systems add a layer of control. They make sure AI gets checked by humans. This helps catch and fix biases caused by bad data.
Importance of Diverse AI Communities
A diverse team is vital for fair AI. Diverse teams can spot and fix biases. Studies show AI can be unfair to certain groups, like women and minorities. Having a diverse team helps make AI fairer for everyone.
Real-World Example | Bias Issue | Mitigation Strategy |
---|---|---|
COMPAS System in Broward County, Florida | Incorrectly labeled African-American defendants as “high-risk” nearly twice as often as white defendants. | Implementing fair assessment criteria and diverse data sets. |
Hiring Algorithm Development | Penalized applicants from women’s colleges. | Ensuring inclusive training data and human oversight. |
“CEO image search” | Only 11% of top results for “CEO” featured women, while women constituted 27% of U.S. CEOs. | Balancing search algorithms to reflect actual diversity statistics. |
Conclusion
Overcoming AI bias requires a multi-faceted strategy to ensure fairness in AI. The complexity of AI bias, from its sources to its social impacts, shows why ethical AI is crucial. Real-life examples, like healthcare underdiagnosis and ChatGPT’s “hallucinations,” highlight the need for action.
Fixing AI bias needs teamwork, strong policies, and new tech. It’s important to involve people from all walks of life in AI development. The Gender Shades project showed big accuracy gaps, especially for darker-skinned women. Tools like predictive policing can make social issues worse.
We must keep working towards ethical AI. Organizations should always look for and fix biases. Our goal is to use AI to improve industries and lives fairly. By tackling AI biases, we can create a fair and inclusive tech world for everyone.
FAQ
What is AI bias and how does it affect society?
AI bias means AI systems show biases we have in society. It makes some groups feel left out. This leads to less trust in tech and keeps old inequalities.
What are some common sources of AI bias?
AI bias comes from biased data, AI’s choices, and how data is labeled. Our own biases and systems also add to the problem.
How does AI bias impact marginalized groups?
It hurts groups already facing challenges by making them doubt tech. It also limits their chances in jobs and society. This can make things worse and hurt our unity.
Can you provide some real-life examples of AI bias?
Sure! In healthcare, data gaps affect diagnosis for different races. Hiring tools might favor certain words. Online ads show jobs differently based on gender. Even image tools show biases in age and gender. And, policing tools might unfairly target races.
What are the economic and social implications of AI bias?
AI bias makes job chances unfair, hurting everyone’s chances. It also makes society more divided. This hurts trust in tech and fairness for all.
How can AI bias be mitigated?
To fix AI bias, we need good AI rules. This means being open, fair, and having humans check things. Using special tech and diverse teams helps too.
Why is AI governance important in addressing AI bias?
Good AI rules make sure tech is fair and open. They help fix bias and build trust. This way, AI helps everyone equally.
How do human-in-the-loop systems help in mitigating AI bias?
These systems add a check by humans. They make sure AI acts fairly and meets our values.
What role do diverse AI communities play in reducing bias?
Diverse teams bring different views. They’re better at spotting and fixing biases. This leads to fairer tech for everyone.
How can technical solutions like counterfactual fairness assessments help in addressing AI bias?
These tools check if AI acts fairly by changing sensitive info. They help find and fix biases, making AI fairer.
Future App Studios is an award-winning software development & outsourcing company. Our team of experts is ready to craft the solution your company needs.